Funnel auto-optimization (AI)
What is an Auto-optimization feature and its specifics?
The auto-optimization feature allows you to set the rules for auto-optimizing your funnel based on the received conversions. When this option is switched on, RedTrack sets the higher weight for a better-converting offer. RedTrack.io AI analyses the funnel paths and sends clicks along with the ones that are highly likely to give the desired results based on accumulated data. An example of the path is the combination of landing and offer or placement (subid value) and conversion.
In other words, you can auto-optimize every X conversions based on the last Y conversions. It means that AI will recalculate how to distribute traffic every X conversions (for example, 10) and will do it based on the historical data of Y (for example, 100). The system will not start the auto-optimization until the number of conversions specified in Y is collected.
How do I apply this feature?
To enable this feature in your account, act as follows:
1. Go to your Campaign settings→ Funnel section→ switch on the Auto optimize toggle→ set up the needed conditions:
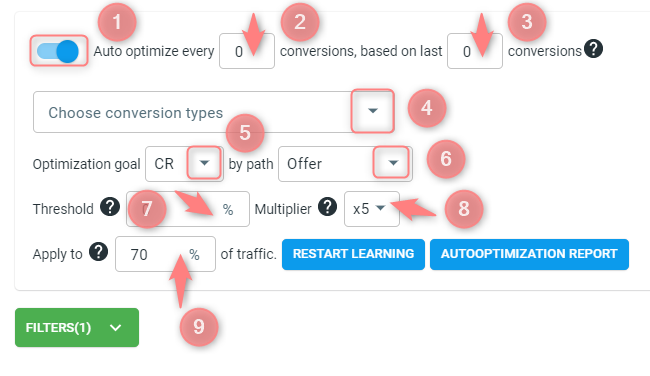
1,2,3 -switch on the toggle and set the needed number of conversions
4 – choose certain conversion types for auto-optimization in this funnel (if needed)
5. Goal – what to check, the metric towards which AI will optimize.
6. Path is the element of the funnel that will contribute performance data towards goal measurement.
☛ Example: CR optimization goal for lander-offer. So AI will analyze the CR for all lander-offer combinations and send most of the traffic to the higher CR, some traffic to the next combination, and so on.
7. Threshold – to become part of the auto-optimization, a particular path should get a threshold goal value or higher.
☛ Example: we have a CR optimization goal for lander-offer. We set the threshold to 1%, which means that any combination of lander-offer that had less than 1% CR will be removed from optimized rotation and will receive some learning traffic (will be explained later). If you use optimization based on subid value. (e.g., placement or publisher) – you can set how many clicks a specific value should receive during the learning period to be included in optimization.
8. Multiplier – defines the subjective bias of the best path value among all winning combinations.
☛ How it works: AI will apply a system of traffic distribution that ensures that all winning combinations get some traffic based on their performance toward the selected goal. You can override this by sending even more traffic to the best path.
9. Apply to – defines how much traffic will be distributed based on performance results. The rest will be distributed randomly based on the funnel setting to feed AI with additional learning data.
As a result, all the paths with the traffic will participate in the optimization (weight will be distributed between them in order to determine where the traffic will go), and these paths will receive a maximum % of the traffic specified in “Apply to”, the rest will be distributed among the other path values that were not included in the optimization.
RedTrack will choose the best path to lead you to the best result. There can be various options (depending on what you choose, the number of paths can be thousands).
2. All the weight changes in the Funnel can be tracked in the Autooptimization report:
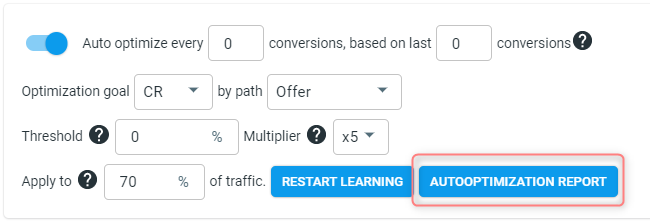
The system will check the number of conversions and the conditions for the funnel:
If you have 4 offers in the funnel and ask to check every 4 conversions based on the last 100, we will start the optimization once the target conversion number is reached (can be 10 for the first offer and 30 for the second, third, and fourth).
We do the optimization based on a path, not a single element. So if a campaign has LP+Offer, then we will optimize this combination as a winning one.
For example:
Landing1-Offer1 – 30
Landing1-Offer2 – 20
Landing2-Offer3 – 10
Landing1-Offer3 – 40
Auto-optimization will start working only after there is enough data based on the rules set.
3. In case you put the wrong values or decided to go the other way, you can always restart the learning by pressing the Restart learning button. The system will start to grab the data for the correct optimization from the beginning:
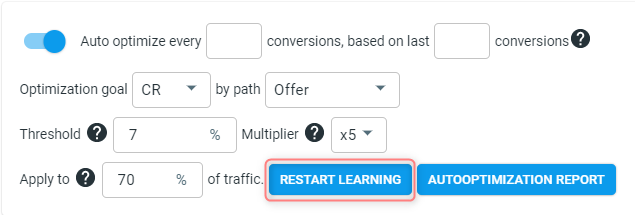